mirror of
https://github.com/sjwhitworth/golearn.git
synced 2025-04-28 13:48:56 +08:00
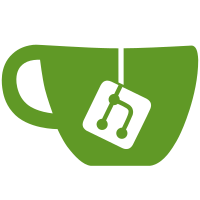
This patch * Adds a one-vs-all meta classifier into meta/ * Adds a LinearSVC (essentially the same as LogisticRegression but with different libsvm parameters) to linear_models/ * Adds a MultiLinearSVC into ensemble/ for predicting CategoricalAttribute classes with the LinearSVC * Adds a new example dataset based on classifying article headlines. The example dataset is drawn from WikiNews, and consists of an average, min and max Word2Vec representation of article headlines from three categories. The Word2Vec model was computed offline using gensim.
54 lines
1.5 KiB
Go
54 lines
1.5 KiB
Go
package linear_models
|
|
|
|
import (
|
|
"fmt"
|
|
"github.com/sjwhitworth/golearn/base"
|
|
)
|
|
|
|
func convertInstancesToProblemVec(X base.FixedDataGrid) [][]float64 {
|
|
// Allocate problem array
|
|
_, rows := X.Size()
|
|
problemVec := make([][]float64, rows)
|
|
|
|
// Retrieve numeric non-class Attributes
|
|
numericAttrs := base.NonClassFloatAttributes(X)
|
|
numericAttrSpecs := base.ResolveAttributes(X, numericAttrs)
|
|
|
|
// Convert each row
|
|
X.MapOverRows(numericAttrSpecs, func(row [][]byte, rowNo int) (bool, error) {
|
|
// Allocate a new row
|
|
probRow := make([]float64, len(numericAttrSpecs))
|
|
// Read out the row
|
|
for i, _ := range numericAttrSpecs {
|
|
probRow[i] = base.UnpackBytesToFloat(row[i])
|
|
}
|
|
// Add the row
|
|
problemVec[rowNo] = probRow
|
|
return true, nil
|
|
})
|
|
return problemVec
|
|
}
|
|
|
|
func convertInstancesToLabelVec(X base.FixedDataGrid) []float64 {
|
|
// Get the class Attributes
|
|
classAttrs := X.AllClassAttributes()
|
|
// Only support 1 class Attribute
|
|
if len(classAttrs) != 1 {
|
|
panic(fmt.Sprintf("%d ClassAttributes (1 expected)", len(classAttrs)))
|
|
}
|
|
// ClassAttribute must be numeric
|
|
if _, ok := classAttrs[0].(*base.FloatAttribute); !ok {
|
|
panic(fmt.Sprintf("%s: ClassAttribute must be a FloatAttribute", classAttrs[0]))
|
|
}
|
|
// Allocate return structure
|
|
_, rows := X.Size()
|
|
labelVec := make([]float64, rows)
|
|
// Resolve class Attribute specification
|
|
classAttrSpecs := base.ResolveAttributes(X, classAttrs)
|
|
X.MapOverRows(classAttrSpecs, func(row [][]byte, rowNo int) (bool, error) {
|
|
labelVec[rowNo] = base.UnpackBytesToFloat(row[0])
|
|
return true, nil
|
|
})
|
|
return labelVec
|
|
}
|