mirror of
https://github.com/sjwhitworth/golearn.git
synced 2025-05-03 22:17:14 +08:00
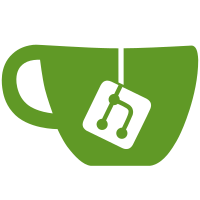
Convergence and LearningRate values less than 0.00, ie. 0.003, were being printed as 0.00 which was incredibly misleading when debugging.
346 lines
9.2 KiB
Go
346 lines
9.2 KiB
Go
package neural
|
|
|
|
import (
|
|
"fmt"
|
|
"github.com/gonum/matrix/mat64"
|
|
"github.com/sjwhitworth/golearn/base"
|
|
"github.com/sjwhitworth/golearn/filters"
|
|
"math"
|
|
"math/rand"
|
|
)
|
|
|
|
// MultiLayerNet creates a new Network which is conceptually
|
|
// organised into layers, zero or more of which are hidden.
|
|
//
|
|
// Within each layer, no neurons are connected.
|
|
//
|
|
// No neurons in a given layer are connected with any neurons
|
|
// in a previous layer.
|
|
//
|
|
// Neurons can only be connected to neurons in the layer above.
|
|
type MultiLayerNet struct {
|
|
network *Network
|
|
attrs map[base.Attribute]int
|
|
layers []int
|
|
classAttrOffset int
|
|
classAttrCount int
|
|
Convergence float64
|
|
MaxIterations int
|
|
LearningRate float64
|
|
}
|
|
|
|
// NewMultiLayerNet returns an underlying
|
|
// Network conceptuallyorganised into layers
|
|
//
|
|
// Layers variable = slice of integers representing
|
|
// node count at each layer.
|
|
func NewMultiLayerNet(layers []int) *MultiLayerNet {
|
|
return &MultiLayerNet{
|
|
nil,
|
|
make(map[base.Attribute]int),
|
|
layers,
|
|
0,
|
|
0,
|
|
0.001,
|
|
500,
|
|
0.90,
|
|
}
|
|
}
|
|
|
|
// String returns a human-readable summary of this network.
|
|
func (m *MultiLayerNet) String() string {
|
|
return fmt.Sprintf("MultiLayerNet(%v, %v, %f, %f, %d", m.layers, m.network, m.Convergence, m.LearningRate, m.MaxIterations)
|
|
}
|
|
|
|
func (m *MultiLayerNet) convertToFloatInsts(X base.FixedDataGrid) base.FixedDataGrid {
|
|
|
|
// Make sure everything's a FloatAttribute
|
|
fFilt := filters.NewFloatConvertFilter()
|
|
for _, a := range X.AllAttributes() {
|
|
fFilt.AddAttribute(a)
|
|
}
|
|
fFilt.Train()
|
|
insts := base.NewLazilyFilteredInstances(X, fFilt)
|
|
return insts
|
|
}
|
|
|
|
// Predict uses the underlying network to produce predictions for the
|
|
// class variables of X.
|
|
//
|
|
// Can only predict one CategoricalAttribute at a time, or up to n
|
|
// FloatAttributes. Set or unset ClassAttributes to work around this
|
|
// limitation.
|
|
func (m *MultiLayerNet) Predict(X base.FixedDataGrid) base.FixedDataGrid {
|
|
|
|
// Create the return vector
|
|
ret := base.GeneratePredictionVector(X)
|
|
|
|
// Make sure everything's a FloatAttribute
|
|
insts := m.convertToFloatInsts(X)
|
|
|
|
// Get the input/output Attributes
|
|
inputAttrs := base.NonClassAttributes(insts)
|
|
outputAttrs := ret.AllClassAttributes()
|
|
|
|
// Compute layers
|
|
layers := 2 + len(m.layers)
|
|
|
|
// Check that we're operating in a singular mode
|
|
floatMode := 0
|
|
categoricalMode := 0
|
|
for _, a := range outputAttrs {
|
|
if _, ok := a.(*base.CategoricalAttribute); ok {
|
|
categoricalMode++
|
|
} else if _, ok := a.(*base.FloatAttribute); ok {
|
|
floatMode++
|
|
} else {
|
|
panic("Unsupported output Attribute type!")
|
|
}
|
|
}
|
|
|
|
if floatMode > 0 && categoricalMode > 0 {
|
|
panic("Can't predict a mix of float and categorical Attributes")
|
|
} else if categoricalMode > 1 {
|
|
panic("Can't predict more than one categorical class Attribute")
|
|
}
|
|
|
|
// Create the activation vector
|
|
a := mat64.NewDense(m.network.size, 1, make([]float64, m.network.size))
|
|
|
|
// Resolve the input AttributeSpecs
|
|
inputAs := base.ResolveAttributes(insts, inputAttrs)
|
|
|
|
// Resolve the output Attributespecs
|
|
outputAs := base.ResolveAttributes(ret, outputAttrs)
|
|
|
|
// Map over each input row
|
|
insts.MapOverRows(inputAs, func(row [][]byte, rc int) (bool, error) {
|
|
// Clear the activation vector
|
|
for i := 0; i < m.network.size; i++ {
|
|
a.Set(i, 0, 0.0)
|
|
}
|
|
// Build the activation vector
|
|
for i, vb := range row {
|
|
if cIndex, ok := m.attrs[inputAs[i].GetAttribute()]; !ok {
|
|
panic("Can't resolve the Attribute!")
|
|
} else {
|
|
a.Set(cIndex, 0, base.UnpackBytesToFloat(vb))
|
|
}
|
|
}
|
|
// Robots, activate!
|
|
m.network.Activate(a, layers)
|
|
|
|
// Decide which class to set
|
|
if floatMode > 0 {
|
|
for _, as := range outputAs {
|
|
cIndex := m.attrs[as.GetAttribute()]
|
|
ret.Set(as, rc, base.PackFloatToBytes(a.At(cIndex, 0)))
|
|
}
|
|
} else {
|
|
maxIndex := 0
|
|
maxVal := 0.0
|
|
for i := m.classAttrOffset; i < m.classAttrOffset+m.classAttrCount; i++ {
|
|
val := a.At(i, 0)
|
|
if val > maxVal {
|
|
maxIndex = i
|
|
maxVal = val
|
|
}
|
|
}
|
|
maxIndex -= m.classAttrOffset
|
|
ret.Set(outputAs[0], rc, base.PackU64ToBytes(uint64(maxIndex)))
|
|
}
|
|
return true, nil
|
|
})
|
|
|
|
return ret
|
|
|
|
}
|
|
|
|
// Fit trains the neural network on the given fixed datagrid.
|
|
//
|
|
// Training stops when the mean-squared error acheived is less
|
|
// than the Convergence value, or when back-propagation has occured
|
|
// more times than the value set by MaxIterations.
|
|
func (m *MultiLayerNet) Fit(X base.FixedDataGrid) {
|
|
|
|
// Make sure everything's a FloatAttribute
|
|
insts := m.convertToFloatInsts(X)
|
|
|
|
// The size of the first layer is the number of things
|
|
// in the revised instances which aren't class Attributes
|
|
inputAttrsVec := base.NonClassAttributes(insts)
|
|
|
|
// The size of the output layer is the number of things
|
|
// in the revised instances which are class Attributes
|
|
classAttrsVec := insts.AllClassAttributes()
|
|
|
|
// The total number of layers is input layer + output layer
|
|
// plus number of layers specified
|
|
totalLayers := 2 + len(m.layers)
|
|
|
|
// The size is then augmented by the number of nodes
|
|
// in the centre
|
|
size := len(inputAttrsVec)
|
|
size += len(classAttrsVec)
|
|
hiddenSize := 0
|
|
for _, a := range m.layers {
|
|
size += a
|
|
hiddenSize += a
|
|
}
|
|
|
|
// Enumerate the Attributes
|
|
trainingAttrs := make(map[base.Attribute]int)
|
|
classAttrs := make(map[base.Attribute]int)
|
|
attrCounter := 0
|
|
for i, a := range inputAttrsVec {
|
|
attrCounter = i
|
|
m.attrs[a] = attrCounter
|
|
trainingAttrs[a] = attrCounter
|
|
}
|
|
m.classAttrOffset = attrCounter + 1
|
|
for _, a := range classAttrsVec {
|
|
attrCounter++
|
|
m.attrs[a] = attrCounter + hiddenSize
|
|
classAttrs[a] = attrCounter + hiddenSize
|
|
m.classAttrCount++
|
|
}
|
|
|
|
// Create the underlying Network
|
|
m.network = NewNetwork(size, len(inputAttrsVec), Sigmoid)
|
|
|
|
// Initialise inter-hidden layer weights and biases to small random values
|
|
layerOffset := len(inputAttrsVec)
|
|
for i := 0; i < len(m.layers)-1; i++ {
|
|
// Get the size of this layer
|
|
thisLayerSize := m.layers[i]
|
|
// Next layer size
|
|
nextLayerSize := m.layers[i+1]
|
|
// For every node in this layer
|
|
for j := 1; j <= thisLayerSize; j++ {
|
|
// Compute the offset
|
|
nodeOffset1 := layerOffset + j
|
|
// For every node in the next layer
|
|
for k := 1; k <= nextLayerSize; k++ {
|
|
// Compute offset
|
|
nodeOffset2 := layerOffset + thisLayerSize + k
|
|
// Set weight randomly
|
|
m.network.SetWeight(nodeOffset1, nodeOffset2, rand.NormFloat64()*0.1)
|
|
}
|
|
}
|
|
layerOffset += thisLayerSize
|
|
}
|
|
|
|
// Initialise biases with each hidden layer
|
|
layerOffset = len(inputAttrsVec)
|
|
for _, l := range m.layers {
|
|
for j := 1; j <= l; j++ {
|
|
nodeOffset := layerOffset + j
|
|
m.network.SetBias(nodeOffset, rand.NormFloat64()*0.1)
|
|
}
|
|
layerOffset += l
|
|
}
|
|
|
|
// Initialise biases for output layer
|
|
for i := 0; i < len(classAttrsVec); i++ {
|
|
nodeOffset := layerOffset + i
|
|
m.network.SetBias(nodeOffset, rand.NormFloat64()*0.1)
|
|
}
|
|
|
|
// Connect final hidden layer with the output layer
|
|
layerOffset = len(inputAttrsVec)
|
|
for i, l := range m.layers {
|
|
if i == len(m.layers)-1 {
|
|
for j := 1; j <= l; j++ {
|
|
nodeOffset1 := layerOffset + j
|
|
for k := 1; k <= len(classAttrsVec); k++ {
|
|
nodeOffset2 := layerOffset + l + k
|
|
m.network.SetWeight(nodeOffset1, nodeOffset2, rand.NormFloat64()*0.1)
|
|
}
|
|
}
|
|
}
|
|
layerOffset += l
|
|
}
|
|
|
|
// Connect input layer with first hidden layer (or output layer
|
|
for i := 1; i <= len(inputAttrsVec); i++ {
|
|
nextLayerLen := 0
|
|
if len(m.layers) > 0 {
|
|
nextLayerLen = m.layers[0]
|
|
} else {
|
|
nextLayerLen = len(classAttrsVec)
|
|
}
|
|
for j := 1; j <= nextLayerLen; j++ {
|
|
nodeOffset := len(inputAttrsVec) + j
|
|
v := rand.NormFloat64() * 0.1
|
|
m.network.SetWeight(i, nodeOffset, v)
|
|
}
|
|
}
|
|
|
|
// Create the training activation vector
|
|
trainVec := mat64.NewDense(size, 1, make([]float64, size))
|
|
// Create the error vector
|
|
errVec := mat64.NewDense(size, 1, make([]float64, size))
|
|
|
|
// Resolve training AttributeSpecs
|
|
trainAs := base.ResolveAllAttributes(insts)
|
|
|
|
// Feed-forward, compute error and update for each training example
|
|
// until convergence (what's that)
|
|
for iteration := 0; iteration < m.MaxIterations; iteration++ {
|
|
totalError := 0.0
|
|
maxRow := 0
|
|
insts.MapOverRows(trainAs, func(row [][]byte, i int) (bool, error) {
|
|
|
|
maxRow = i
|
|
// Clear vectors
|
|
for i := 0; i < size; i++ {
|
|
trainVec.Set(i, 0, 0.0)
|
|
errVec.Set(i, 0, 0.0)
|
|
}
|
|
|
|
// Build vectors
|
|
for i, vb := range row {
|
|
v := base.UnpackBytesToFloat(vb)
|
|
if attrIndex, ok := trainingAttrs[trainAs[i].GetAttribute()]; ok {
|
|
// Add to Activation vector
|
|
trainVec.Set(attrIndex, 0, v)
|
|
} else if attrIndex, ok := classAttrs[trainAs[i].GetAttribute()]; ok {
|
|
// Set to error vector
|
|
errVec.Set(attrIndex, 0, v)
|
|
} else {
|
|
panic("Should be able to find this Attribute!")
|
|
}
|
|
}
|
|
|
|
// Activate the network
|
|
m.network.Activate(trainVec, totalLayers-1)
|
|
|
|
// Compute the error
|
|
for a := range classAttrs {
|
|
cIndex := classAttrs[a]
|
|
errVec.Set(cIndex, 0, errVec.At(cIndex, 0)-trainVec.At(cIndex, 0))
|
|
}
|
|
|
|
// Update total error
|
|
totalError += math.Abs(errVec.Sum())
|
|
|
|
// Back-propagate the error
|
|
b := m.network.Error(trainVec, errVec, totalLayers)
|
|
|
|
// Update the weights
|
|
m.network.UpdateWeights(trainVec, b, m.LearningRate)
|
|
|
|
// Update the biases
|
|
m.network.UpdateBias(b, m.LearningRate)
|
|
|
|
return true, nil
|
|
})
|
|
|
|
totalError /= float64(maxRow)
|
|
// If we've converged, no need to carry on
|
|
if totalError < m.Convergence {
|
|
break
|
|
}
|
|
}
|
|
}
|